Coordinated Expression Domains in Mammalian Genomes
Description
Identifying the genes underlying quantitative trait loci (QTL) for disease has proven difficult, mainly due to the low resolution of the approach and the complex genetics involved. However, recent advances in bioinformatics and the availability of genetic resources now make it possible to narrow the genetic intervals and test candidate genes. In addition to identifying the causative genes, defining the pathways that are affected by these QTL is of major importance as it can give us insight into the disease process and provide evidence to support candidate genes.
In the first study (Hageman et al. J Am Soc Nephrol. 2011), we mapped three significant and one suggestive QTL on Chromosomes (Chrs) 1, 4, 15, and 17, respectively, for increased albumin excretion (measured as albumin-to-creatinine ratio) in a cross between the MRL/MpJ and SM/J mouse inbred strains. By combining data from several sources and by utilizing gene expression data, we identified Tlr12 as a likely candidate for the Chr 4 QTL. Through the mapping of 33,881 transcripts measured by microarray on kidney RNA from each of the 173 male F2 animals, we identified several downstream pathways associated with these QTL. Among these were the glycan degradation, leukocyte migration, and antigen presenting pathways. We demonstrate that by combining data from multiple sources, we can identify not only genes that are likely to be causal candidates for QTL, but also the pathways through which these genes act to alter phenotypes. This combined approach provides valuable insights into the causes and consequences of renal disease. Female MRL/MpJ (MRL) mice were crossed with male SM/J (SM) mice; their progeny were intercrossed to produce 371 F2 animals. Only 173 F2 males were used for this study.
In the second study (Hageman et al. Genetics 2011), we proposed a Bayesian statistical method to infer networks of causal relationships among genotypes and phenotypes using expression quantitative trait loci (eQTL) data from genetically randomized populations. Causal relationships between network variables are described with hierarchical regression models. Prior distributions on the network structure enforce graph sparsity and have the potential to encode prior biological knowledge about the network. An efficient Monte Carlo method is used to search across the model space and sample highly probable networks. The result is an ensemble of networks that provide a measure of confidence in the estimated network topology. These networks can be used to make predictions of system-wide response to perturbations. We applied our method to kidney gene expression data from the MRL/MpJ × SM/J intercross population and predicted a previously uncharacterized feedback loop in the local renin-angiotensin system.
Data
GEO DataSets
GSE23310
QTL Archive data
hageman_2011
Graphical Modeling Codes (v02):
README (.docx)
Folder of codes (.zip)
Publications
Hageman RS, Leduc MS, Caputo CR, Tsaih SW, Churchill GA, Korstanje R
J Am Soc Nephrol. 2011 Jan;22(1):73-81 [ Full Text ] PMCID: PMC3014036
[ MPD data ]
Hageman RS, Leduc MS, Korstanje R, Paigen B, Churchill GA
Genetics. 2011 Apr;187(4):1163-70 [ Full Text ] PMCID: PMC3070524
[ MPD data ]
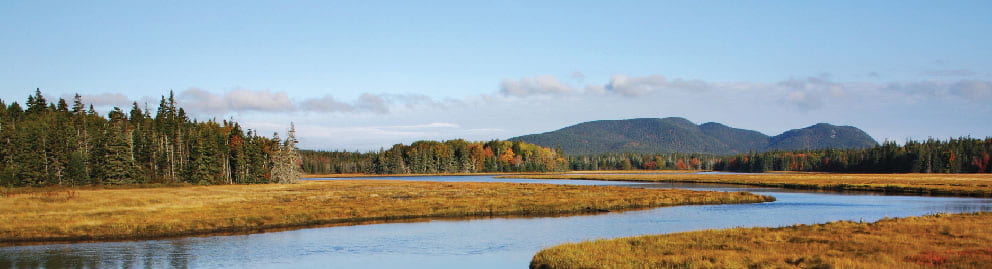