Summer Students
Sarah Benjamin - Maine School of Science & Math, Limestone, ME - 2009
Research Project at The Churchill Lab
Type II diabetes is a disease that affects over 17.9 million people in the United States alone. The goal of this study is to develop a gene expression model that shows the relationships between specific genes in the liver, and how they affect the phenotypes insulin and glucose. The first part of my project involved analyzing all the transcript data for liver tissue that I was given. I used R, a statistical software, to identify quantitative trait loci, or regions of the genome that have a strong relationship with the clinical trait in question, and correlated the transcripts with insulin and glucose. This produced a gene list that I was then able to use to produce a graphical model showing how these genes interact with each other. I identified chromosomes 1,17, and 6 as chromosomes of interest using QTL analysis of insulin and glucose. I also identified approximately forty genes of interest. The next step in this project is to map these genes to the insulin signaling pathway to determine their effect on insulin resistance, and identify strong candidates that can be linked to the direct cause of insulin resistance and type II diabetes.
Marion Elizabeth Deerhanke - The North Carolina School of Science and Mathematics, Durham, NC - 2008
Elizabeth investigated hypertension as a complex phenotype and searched for the genetic basis of this widespread disease using quantitative trait loci analysis. In her study she conducted a QTL analysis of systolic blood pressure in F2 males from eight intercrosses comprising fourteen inbred mouse strains. The use of multiple crosses allows for greater precision in narrowing QTL regions through identification of concordant peaks. The search for individual and interacting pairs of loci affecting systolic blood pressure indicated fourteen significant QTL, three epistatic interactions, and linked QTL on Chr 3. Through multiple regression analysis, she developed multiple QTL models for each of the eight intercrosses accounting for as much as 35% of phenotypic variance. These novel loci affecting blood pressure contributed to our understanding of the complex genetic basis of hypertension.
Jasmine Johnson - 2012
Identification of a Genetic Network Linking Sleep and Adiposity
Obesity is one of the most detrimental health problems of the 21st century. Over the past few decades, the obesity epidemic has become a major public health problem of the 21st century. In 2008, approximately 500 million adults were diagnosed as obese. By 2015, scientists predict that 700 million adults will be diagnosed as obese in America (World Health Organization, 2011). Past studies have shown that there may be a relationship between amount of sleep and adipose tissue, but the intricacies of this relationship have not been thoroughly studied (University of Chicago Medical Center, 2010). Circadian rhythms, controlled by the suprachiasmatic nucleus in the brain, control both the sleep-wake cycle and the feeding cycle and may be the underlying mechanism linking sleep to adipose tissue (Laposky et al, 2007). A method for studying underlying mechanisms, Quantitative Trait Loci analysis , will be conducted on gene expression data from the different regions of the brain and multiple sleep-wake traits from the B6/BALB cross in order to determine the varying relationships between the sleep-wake traits in addition to relationships between both the expression data and the sleep traits. After linkage analysis has been conducted, significant genes identified through the gene expression data as well MGI will be used to create a genetic network linking adiposity and metabolic genes to sleep QTL Preliminary results show that chromosomes 5 and 13 may be chromosomes of interest in the phenotypic data. Using the confidence intervals of chromosome 13 in the phenotypic data, 600 genes have been identified for chromosome 13 using MGI. In the expression data , there were 120 significant genes with 5 of these genes overlapping between the two. Further analysis of the relationship between the genes and sleep-wake traits will be conducted using conditional genome scans. Additionally, we will strategic equation mapping analysis between to the sleep-wake traits to determine any causal, reactive or independent relationships.
Samuel Elliot Krause - The Jackson Laboratory 600 Main Street Bar Harbor Maine, 04609 - 2016
I examined the relationship between aging and immune cells in the Diversity Outbred mice.
Renée Symonds- Bowdoin College, Brunswick, ME - 2010
Text about the project.Natural variation is an important component underlying multiple traits of medical and commercial relevance. The objective of this study was to use genotype and microarray data to predict unobserved phenotypes. Methodology/Principal Findings: Linear models representing trait networks were built in soybeans (Glycine max) from the training dataset, which consists of genotype data at 941 markers and microarray data for 28,395 genes. The approach used natural genetic variation to infer statistical models of complex networks for genetic and genomic data. Numerous models that incorporate genotype data, microarray data, and a combination of both data sets were estimated to predict complex disease phenotypes related to a major soybean pathogen (Phytophthora sojae). Linear Models were adjusted by two methods: the least squares regression and a regularized multivariate regression approach called Elastic Net. Their predictive ability was assessed by 10-fold cross validation to measure prediction. For genotype data, QTL analysis followed by step-wise variable selection outperformed Elastic Net in terms of the prediction accuracy of the two phenotypes (Err=0.823, 0.670 vs. Err=1.00, 0.982). For microarray data, Elastic Net produced the most predictive model (0.332 and 0.327 vs. 1.210 and 0.980). Combining genotype and microarray data showed that Elastic Net produces the best predictive model for Phenotype 1, while linear modeling produced the best model for Phenotype 2 (Err=0.926, 0.982 vs. Err=1.79, 0.769). Results were consistent for two disease resistance phenotypes analyzed separately. Conclusions: Genome-wide gene expression data best captures information about genetic effects on disease resistance in soybean. The model was not improved by the addition of QTL genotypes in the model.
Gabe Vela - Rockdale Magnet School of Science and Technology, Conyers, GA - 2012
Identification of QTL and Candidate Genes Underlying Sleep
Sleep, a fundamental behavior that occupies about one-third of the human lifespan, has been linked to various physical and behavioral diseases such as obesity or metabolic syndrome. While there is much known about sleep-wake properties, little is known about the identity or nature individual of genes behind sleep regulation. We have set out to identify individual genes that regulate sleep traits in order to further our understanding of the mechanisms underlying sleep and its effect on our bodies. We performed quantitative trait locus (QTL) mapping using the R statistical programming language in order to determine regions of the genome that influence sleep traits. Results from the analysis revealed a large number of sleep QTLs on chromosome 17. We then set out to find genes that shared a QTL with sleep traits, and to correlate expression data from said genes with sleep traits using R in order to compile a list of genes that regulate the sleep QTLs on chromosome 17. We identified Lta, a gene known to be a cause of abnormal sleep patterns, and Twsg1, which may be further researched as a gene involved in sleep mechanisms.
David Witmer - The Maine School of Science and Mathematics, Limestone, ME - 2007
David investigated gene expression patterns in multi-factorial DNA microarray data. The core of his study was the utilization of ANOVA-based statistical tests to test general and focused research hypotheses through overall F-tests and specific contrasts. Groups of co-expressed genes were resolved through hierarchical and k-means clustering analysis. Important biological processes associated with key factors were determined by statistical tests for association with gene ontology (GO) terms. Finally, he investigated relationships between gene expression levels and phenotypic response patterns.
Luis Zapata - North Carolina School of Science and Math - 2006
Luis worked on analyzing, quantifying, and assessing the gene expression in 12 inbred mouse strains of each sex raised on high fat or chow diets using Genechip arrays. Luis assessed probe level quality, normalized probe intensity, adjusted background, and performed graphical diagnostics on microarray images. He then assessed the influence and interactions of strain, sex, and diet on the overall intensity value of each gene on the array. He finally generated lists of genes that will be studied for functional associations using available genomic annotation software tools.
Alan Bohn - 2009 North Carolina School of Science & Math, Durham, NC - 2009
Research Project at The Churchill Lab
NSAIDs such as ibuprofen are commonly used to control inflammation byinhibiting COX-2. However, the side effects of COX-2 inhibition not fully understood. Transcriptional effects of COX-2 inhibition were explored by comparing the liver and adipose tissues of genetically altered COX-1 > COX-2 exchange mice (B6.129(FVB)-Ptgs2tm2.1(Ptgs1)Fun/J) to C57BL/6NJ controls. ANOVA models were used to identify differentially expressed genes between strains for each tissue. Significantly enriched pathways were determined using Gene Set Enrichment information. GenMapp was used to visualize enriched pathways and patterns of differentially expressed genes. Results show no significant difference in the Ptgs1 or the Ptgs2 locus between the COX-1 > COX-2 mice and the controls, nor are there downstream consequences in prostaglandin synthesis. Results indicate up-regulation in the cholesterol biosynthesis pathway in both liver and adipose tissues. Liver tissue has up-regulated bile acid metabolism and down-regulated gluconeogenesis. In the Reverse Cholesterol Transport pathway, Scarb (SR-B1) and Cel are significantly up-regulated. This suggests an increase in selective uptake of cholesterol esters from HDL. In adipose tissue, there is up-regulation in fatty acid metabolism, ketone degradation, and glycolysis. Therefore, several pathways that produce Acetyl-CoenzymeA are up-regulated, yet Cholesterol Biosynthesis is the only enriched pathway that uses Acetyl-CoenzymeA as a substrate.
Justin Huang - North Carolina School of Science & Math, Durham, NC - 2009
Many cases of obesity are caused by sedentary lifestyle, but research has also shown that there is a significant genetic factor in the onset of obesity. The goal of this study was to use a Systems Biology approach to identify candidate genes causing obesity in mice that can be tested as drug targets of the treatment of obesity. Quantitative Trait Loci (QTL) analysis was complemented with genome-wide gene expression profiling to discover networks of gene co-expression that are associated to the Fat Percentage (FP) phenotype in an F2 cross between the C57BL/6J and C3H/HeJ mouse strains. An over-representation test was performed to identify biochemical pathways that are over-represented with genes with high correlation to FP and that share one or more QTL with this phenotype. Co-expression networks were then enriched with positional candidates genes that were members of the most significant pathways. Networks were also enriched with genes with LOD score profile highly similar to FP. As a result, I propose Crla2 and Icos as best candidates for the FP QTL on chromosome 1 and the topology of the co-expression networks suggest Rab27b and Sult1e1 as best candidates for drug manipulation for the treatment of obesity.
Ryan Keating - The Maine School of Science and Mathematics, Limeston, ME - 2008
Ryan investigated chronic kidney disease (CKD). CKD, a complex trait, is affected by developmental, environmental, and genetic factors. In his study, eight intercrosses from fourteen inbred mouse strains were analyzed for genetic factors that influence CKD. He performed quantitative trait loci (QTL) analysis of kidney weight with a covariate of body weight to identify regions of the mouse genome that affect CKD. From these eight crosses, he identified twenty significant (P <.05) QTL and eight interactive QTL pairs and accounted for variance in kidney weight, ranging from 51.7 to 73.0 percent. Identification of candidate genes from the significant QTL could then be used to locate orthologous regions in humans.
Lauren Reagin - Rockdale Magnet School for Science & Technology, Conyers, GA - 2011
QTL Mapping Analysis of a Diabetic Mouse Backcross (NZO x NON)
There are 23.6 million people that have diabetes within the United States (ADA, 2007). Previous studies have shown a genetic link to this devastating disease. The main purpose of this experiment is to identify QTL’s (quantitative trait loci) that have an effect on the phenotypes of diabetes and to make graphical models. The phenotypic effects of diabetes include leptin resistance, insulin resistance, and high glucose levels. Also, often obesity and diabetes are correlated with each other, so body weight and the fat pad traits (mesenteric, inguinal, peritoneal, and gonadal fat) are used as phenotypes. The research hypothesis: There is a correlation that can be found between the phenotypes and QTL’s found in the data set and a graphical model can be made. The analysis of the data set uses a statistical, code-based program called R, using the r/QTL package, which allows genetic data to be analyzed. Using the results from the analysis, two separate models have been crafted. One model is over the interaction between the fat pad traits, leptin, and the QTL’s. The second model is over body weight and the fat pad traits with their interactions with glucose and the QTL’s.
Casey Thornton - Maine School of Science & Math, Limestone - 2011
Quantitative Trait Loci analysis of insulin and gene expression in pancreatic ß-cells in a BTBRxB6BL/6J intercross
Previous research indicates that chronic activation of endoplasmic reticulum (ER) stress leads to insulin resistance and diabetes in obesity. My objective is to continue this research by developing graphical models for the type II diabetes related ER stress response pathway. This correlates specific genes in the pancreas to how they affect the clinical phenotypes insulin, leptin and LIF (Leukemia Inhibitory Factor). QTL analysis was conducted on 40,574 transcripts though my research narrowed to 13 candidate genes of interest that were derived from ER stress literature. To do this I used R, a command prompt open source statistical software console. In R, QTL, or Quantitative Trait Loci, analysis determines the extent to which a region of the genome influences a quantitative phenotype. In addition, chromosomes 2, 5, 6, 16, and 19 were marked as QTL of interest for the clinical phenotypes insulin, leptin and LIF. BIC (Bayesian Information Criterion) analysis was then used in the Rqtl package as a predictor of likely causal models that relate the QTL, genes, and clinical phenotypes. From this mapping I was able to identify likely candidate relationships that can be linked to the direct cause of insulin resistance in type II diabetes.
Madeline Williams - The Jackson Laboratory 600 Main Street Bar Harbor Maine, 04609 - 2016
Kali Xu - North Carolina School of Science & Math, Durham, NC - 2011
Quantitative trait loci analysis of the relationship between atherosclerotic indicators and gene expression in an MRL/MpJxSM/J F2 intercross
Atherosclerosis is a chronic disease deriving from a combination of inflammatory and lipid metabolism pathways. It is a precursor of cardiovascular disease, the number one cause of death and disability in North America. This study evaluated the genetic factors underlying variation in key atherosclerotic risk factors such as total cholesterol. Using the statistical software R, I conducted a quantitative trait loci (QTL) analysis of liver genotype, phenotype, and transcript data from an MRL/MpJxSM/J F2 mouse intercross. This analysis yielded cholesterol QTL peaks on chromosomes 1, 4, 7, and 18, and after examining the QTL confidence interval on chromosome 18 for cis expression QTL, I found five transcripts to be significant in regulating this QTL. I created linear regression models for these gene, phenotype, and QTL relationships based on the results of Bayesian information criterion analysis and then built a graphical model to represent these relationships. I confirmed one previously known candidate gene affecting cholesterol level (Apoa2) and identified a new candidate gene (Isoc1) whose role in cholesterol regulation may be further explored in future studies. I also found a relationship between cholesterol and bone mineral density that, if validated, may bring up interesting new implications for cholesterol lowering treatments.
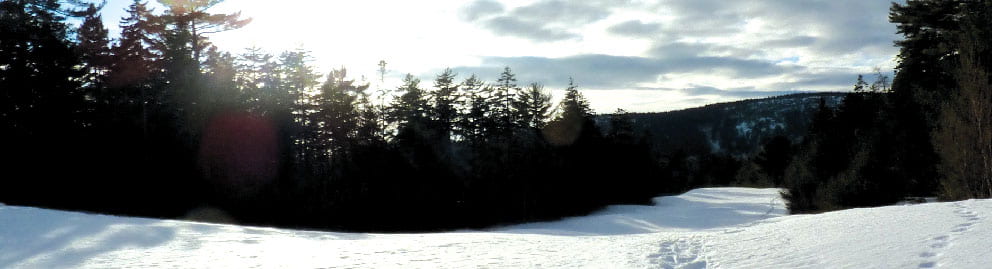